Influence of parameters:
Number of measured values - n_cycles (P-AXIS-00413):
The parameter P-AXIS-00413 specifies the number of measured values that are included in the prediction model of the moving averaging filter. Accordingly, the larger the number of included measured values, the better the smoothing effect. The prediction characteristic of the Kalman filter reduces the dead time compared to a conventional moving averaging filter. However, it should be noted that the dead time of the prediction model leads to an oscillation at large changes in distance. The distance increases as the number of included measured values rises (P-AXIS-00413).
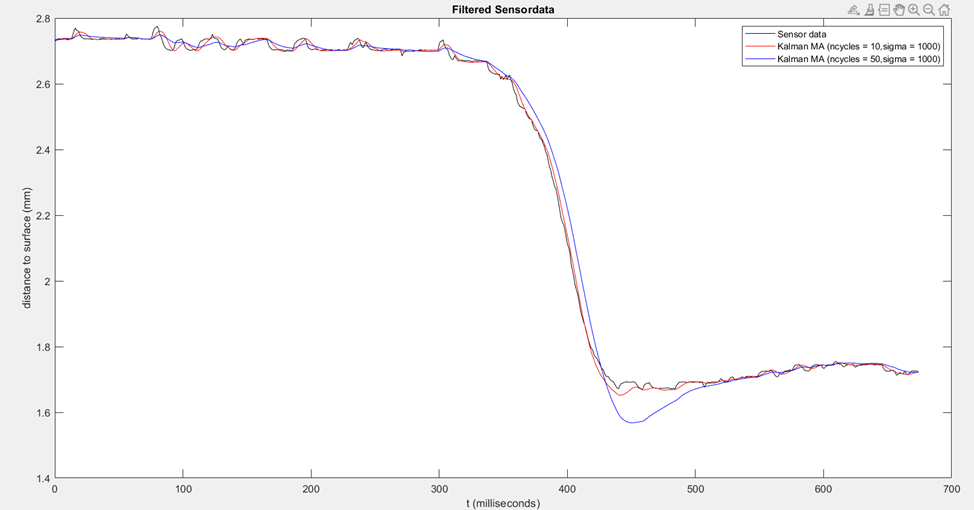
Degree of uncertainty - sigma (P-AXIS-00783):
The parameter P-AXIS-00783 indicates the degree of uncertainty of the recorded measured values. The lower the specified uncertainty of the measured values, the more the prediction from the moving averaging filter is approximated to the actual measured values.
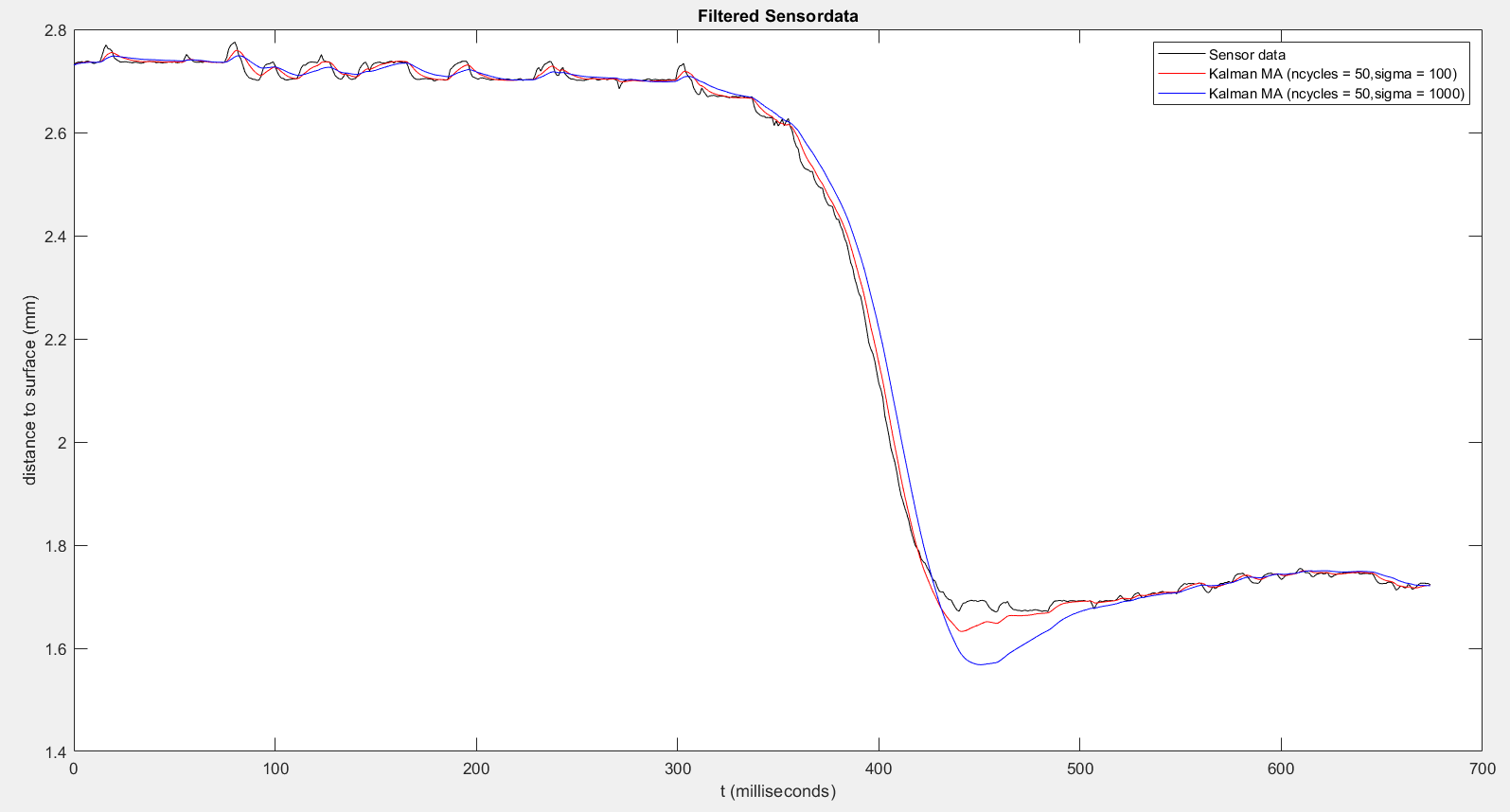
Example
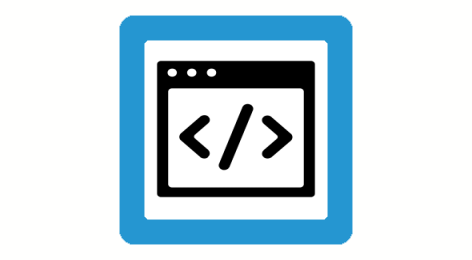
Example parameters: Kalman filter with averaging filter model
kenngr.distc.filter_type KALMAN_MA #Filter type
kenngr.distc.n_cycles 30 #Number of included measured values
kenngr.distc.kalman_sigma 1000 #Uncertainty of measured values